P7.005. Final : Xu hướng Công nghệ 2018 - AGI, Quantum Computing
Phần 5 của loạt bài viết về Xu hướng Công nghệ 2018 gồm có: 8. Công nghệ Lũy thừa: AGI, Quantum Computing 9. Cảm ơn Tác giả ...
Phần 5 của loạt bài viết về Xu hướng Công nghệ 2018 gồm có:
8. Công nghệ Lũy thừa: AGI, Quantum Computing
9. Cảm ơn Tác giả

Xem Báo cáo Công nghệ 2018
Tóm tắt,
8. Danh sách theo dõi Công nghệ Lũy thừa: Chân trời Cơ hội Đổi mới
the promise—and challenge—of artificial general intelligence and quantum computing, with major ramifications for security and much more.
response to these and other disruptive forces, creating capabilities to sense, scan, vet, experiment, incubate, and scale.
SCIENCE author Steven Johnson once observed that “innovation doesn’t come just from giving people incentives; it comes from creating environments where their ideas can connect.
These are emerging technology forces that we think could manifest in a “horizon 3 to 5” timeframe—between 36 and 60 months. With some exponentials, the time horizon may extend far beyond five years before manifesting broadly in business and government. For example, artificial general intelligence (AGI) and quantum encryption, which we examine later in this chapter, fall into the 5+ category.
Now is the time to begin constructing an exponentials innovation environment in which, as Johnson says, “ideas can connect.”
t that time, these emerging technologies were outpacing Moore’s Law: Their performance relative to cost (and size) was more than doubling every 12 to 18 months. Just a few years later, we see these same technologies are disrupting industries, business models, and strategies.
Some efforts will focus on core innovation that optimizes existing products for existing customers. Others are around adjacent innovation that can help expand existing markets or develop new products working from their existing asset base. Others still target transformational innovation—that is, deploying capital to develop solutions for markets that do not yet exist or for needs that customers may not even recognize that they have.
A striking pattern emerged: Outperforming firms typically allocate about 70 percent of their innovation resources to core offerings, 20 percent to adjacent efforts, and 10 percent to transformational initiatives. In contrast, cumulative returns on innovation investments tend to follow an inverse ratio, with 70 percent coming from the transformational initiatives, 20 percent from adjacent, and 10 percent from core
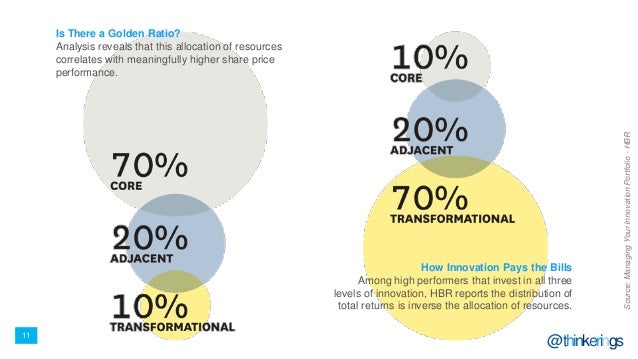
truly disruptive technologies are often deployed first to improve existing products and processes—that is, those in the core and nearby adjacent zones. Only later do these technologies find net new whitespace applications.
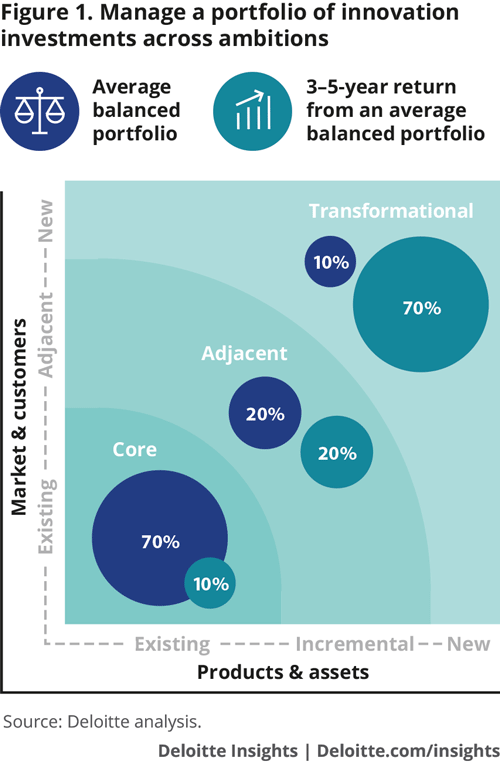
PURSUING THE “UNKNOWABLE”
As you begin planning the exponentials innovation journey ahead, consider taking a lifecycle approach that includes the following steps:
- Sensing and research. As a first step, begin building hypotheses based on sensing and research. Identify an exponential force and hypothesize its impact on your products, your production methods, and your competitive environment in early and mid-stage emergence.
- Exploration and experimentation. At some point, your research reaches a threshold at which you can begin exploring the “state of the possible.” Look at how others in your industry are approaching or even exploiting these forces. collect 10 or more exemplars of what others are doing with exponentials. developing an ecosystem around each exponential force could help you engage external business partners, vendors, and suppliers as well as stakeholders in your own organization. exploring “state of the practical.” Specifically, which elements of a given exponential force can potentially benefit the business? prioritize use cases, develop basic business cases, and then build initial prototypes.
- Incubation and scaling. When the value proposition of the experiment meets the expectations set forth in your business case, you may be tempted to put the innovation into full enterprise-wide production.
- Be programmatic: Taking any innovation—but particularly one grounded in exponential forces—from sensing to production is not a two-step process, nor is it an accidental process. Inspiration is an ingredient, but so is perspiration.
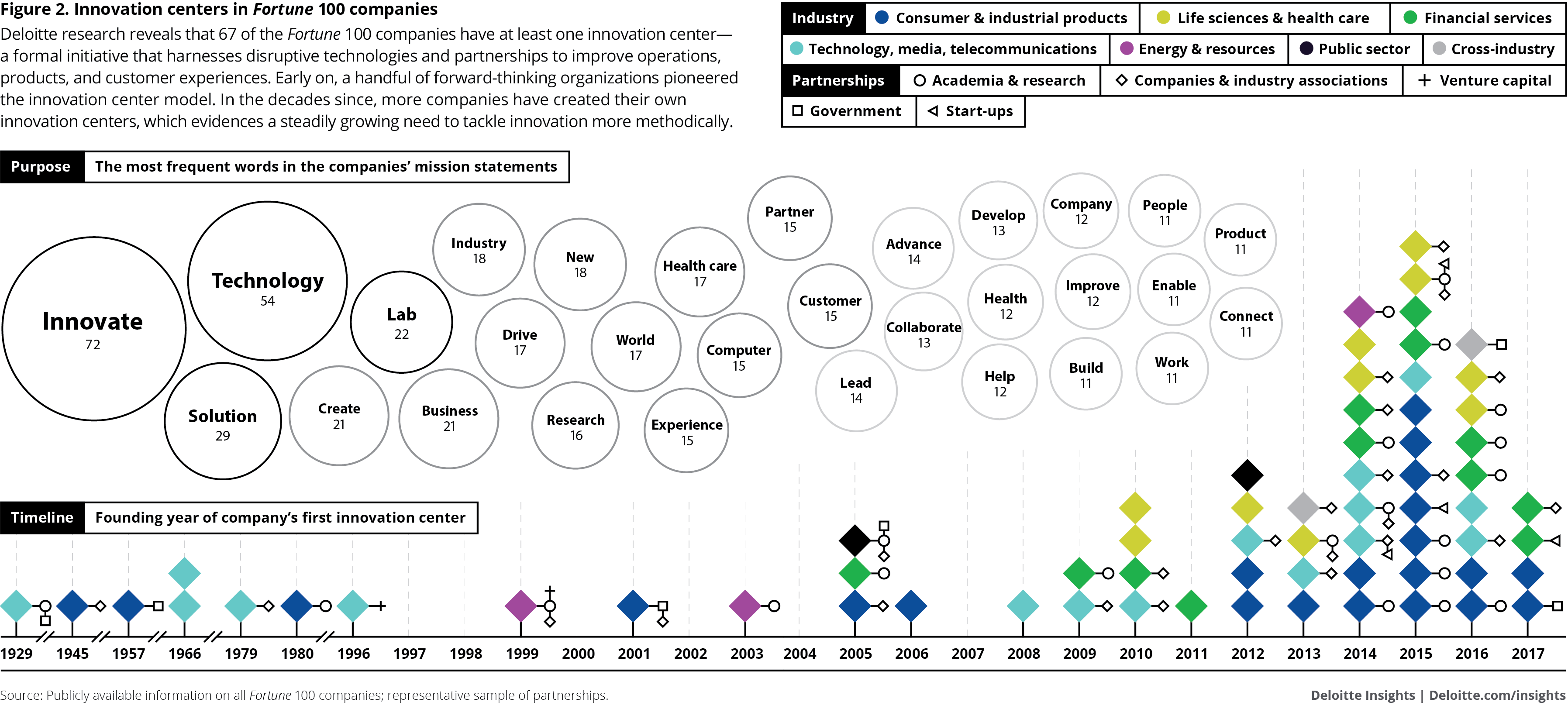
DON’T FORGET THE HUMANS
As you dive into exponentials and begin thinking more deliberately about the way you approach innovation, it is easy to become distracted or discouraged.
human beings are the fundamental unit of economic value. For example, people remain at the center of investment processes, and they still make operational decisions about what innovations to test and deploy.
Our take
JONATHAN KNOWLES, HEAD OF FACULTY AND DISTINGUISHED FELLOW
PASCAL FINETTE, VICE PRESIDENT OF STARTUP SOLUTIONS
SINGULARITY UNIVERSITY
PASCAL FINETTE, VICE PRESIDENT OF STARTUP SOLUTIONS
SINGULARITY UNIVERSITY
Humans are not wired to think in an exponential way.
Yet exponential progress is happening, especially in technologies. Consider this very basic example: In 1997, the $46 million ASCI Red supercomputer had 1.3 teraflops of processing power, which at the time made it the world’s fastest computer.5 Today, Microsoft’s $499 Xbox One X gaming console has 6 teraflops of power.6 Mira, a supercomputer at Argonne National Laboratory, is a 10 petaflop machine.7 That’s ten thousand trillion floating point operations per second!
Exponential innovation is not new, and there is no indication it will slow or stop.
Artificial general intelligence
In the 2013 Spike Jonze film Her, a sensitive man on the rebound from a broken marriage falls in love with “Samantha,” a new operating system that is intuitive, self-aware, and empathetic.
Consider the disruptive potential of a fully realized AGI solution: Virtual marketers could analyze massive stores of customer data to design, market, and sell products and services—data from internal systems fully informed by social media, news, and market feeds. Algorithms working around the clock could replace writers altogether by generating factual, complex, situation-appropriate content free of biases and in multiple languages. This list goes on.
AI’s current strength lies primarily in “narrow” intelligence—so-called artificial narrow intelligence (ANI), such as natural language processing, image recognition, and deep learning to build expert systems. A fully realized AGI system will feature these narrow component capabilities, plus several others that currently do not yet exist: the ability to reason under uncertainty, to make decisions and act deliberately in the world, to sense, and to communicate naturally.
TALKIN’ ’BOUT AN EVOLUTION
During the next three to five years, expect to see improvements in AI’s current component capabilities.
What you probably won’t see in this time horizon is the successful development, integration, and deployment of all AGI component capabilities. We believe that milestone is at least 10+ years away.
applications for ANI components, such as pattern recognition to diagnose skin cancer, or machine learning to improve decision-making in HR, legal, and other corporate functions.
The state-of-the-art reflects progress in each sub-problem and innovation in pair-wise integration. Vision + empathy = affective computing. Natural language processing + learning = translation between languages you’ve never seen before. Google Tensor Flow may be used to build sentiment analysis and machine translation, but it’s not easy to get one solution to do both well. Generality is difficult. Advancing from one domain to two is a big deal; adding a third is exponentially harder.
John Launchbury, former director of the Information Innovation Office at the Defense Advanced Research Projects Agency, describes a notional artificial intelligence scale with four categories: learning within an environment; reasoning to plan and to decide; perceiving rich, complex, and subtle information; and abstracting to create new meanings
the first wave of AI as handcrafted knowledge in which humans create sets of rules to represent the structure of knowledge in well-defined domains, and machines then explore the specifics. These expert systems and rules engines are strong in the reasoning category and should be important elements of your AI portfolio.
the second wave—which is currently under way—as statistical learning. In this wave, humans create statistical models for specific problem domains and train them on big data with lots of label data, using neural nets for deep learning. These second-wave AIs are good at perceiving and learning but less so at reasoning.
the next wave as contextual adaptation. In this wave, AI constructs contextual explanatory models for classes of real-world phenomena; these waves balance the intelligence scale across all four categories, including the elusive abstracting.
Machine learning, paired with emotion recognition software, has demonstrated that it is already at human-level performance in discerning a person’s emotional state based on tone of voice or facial expressions
Though it made hardly a ripple in the press, the most significant AGI breadcrumb appeared on January 20, 2017, when researchers at Google’s AI skunkworks, DeepMind, quietly submitted a paper on arXiv titled “PathNet: Evolution Channels Gradient Descent in Super Neural Networks.” While not exactly beach reading, this paper will be remembered as one of the first published architectural designs for a fully realized AGI solution.
My take
OREN ETZIONI, CEO
ALLEN INSTITUTE FOR ARTIFICIAL INTELLIGENCE
ALLEN INSTITUTE FOR ARTIFICIAL INTELLIGENCE
In March 2016, the American Association for Artificial Intelligence and I asked 193 AI researchers how long it would be until we achieve artificial “superintelligence,” defined as an intellect that is smarter than the best human in practically every field. Of the 80 Fellows responding, roughly 67.5 percent of respondents said it could take a quarter century or more. 25 percent said it would likely never happen.
you don’t have to wait for AGI to appear (if it ever does) to begin exploring AI’s possibilities. Some companies are already achieving positive outcomes with so-called artificial narrow intelligence (ANI) applications by pairing and combining multiple ANI capabilities to solve more complex problems.
natural language processing integrated with machine learning can expand the scope of language translation; computer vision paired with artificial empathy technologies can create affective computing capabilities. Consider self-driving cars, which have taken the sets of behaviors needed for driving—such as reading signs and figuring out what pedestrians might do—and converted them into something that AI can understand and act upon.
the journey from ANI to AGI is not just difference in scale. It requires radical improvements and perhaps radically different technologies.
Quantum encryption: Endangered or enabled?
in the future—perhaps within a decade—quantum computers that are exponentially more powerful than the most advanced supercomputers in use today could help address real-world business and governmental challenges
In fact, work on developing post-quantum encryption around some principles of quantum mechanics is already under way.
new encryption techniques to “quantum-proof” information—including techniques that do not yet exist. There are, however, several interim steps organizations can take to enhance current encryption techniques and lay the groundwork for additional quantum-resistant measures as they emerge.
UNDERSTANDING THE QUANTUM THREAT
there is an active race under way to achieve a state of “quantum supremacy” in which a provable quantum computer surpasses the combined problem-solving capability of the world’s current supercomputers.
Shor’s algorithm. In 1994, MIT mathematics professor Peter Shor developed a quantum algorithm that could factor large integers very efficiently. The only problem was that in 1994, there was no computer powerful enough to run it. Even so, Shor’s algorithm basically put “asymmetric” cryptosystems based on integer factorization—in particular, the widely used RSA—on notice that their days were numbered
To descramble encrypted information—for example, a document or an email—users need a key. Symmetric or shared encryption uses a single key that is shared by the creator of the encrypted information and anyone the creator wants to access the information. Asymmetric or public-key encryption uses two keys—one that is private, and another that is made public. Any person can encrypt a message using a public key. But only those who hold the associate private key can decrypt that message. With sufficient (read quantum) computing power, Shor’s algorithm would be able to crack two-key asymmetric cryptosystems without breaking a sweat. It is worth noting that another quantum algorithm—Grover’s algorithm, which also demands high levels of quantum computing power—can be used to attack ciphers.24
One common defensive strategy calls for larger key sizes. However, creating larger keys requires more time and computing power. Moreover, larger keys often result in larger encrypted files and signature sizes. Another, more straightforward post-quantum encryption approach uses large symmetric keys. Symmetric keys, though, require some way to securely exchange the shared keys without exposing them to potential hackers. How can you get the key to a recipient of the encrypted information? Existing symmetric key management systems such as Kerberos are already in use, and some leading researchers see them as an efficient way forward. The addition of “forward secrecy”—using multiple random public keys per session for the purposes of key agreement—adds strength to the scheme. With forward secrecy, hacking the key of one message doesn’t expose other messages in the exchange.
Key vulnerability may not last indefinitely. Some of the same laws of quantum physics that are enabling massive computational power are also driving the growing field of quantum cryptography. In a wholly different approach to encryption, keys become encrypted within two entangled photons that are passed between two parties sharing information, typically via a fiber-optic cable. The “no cloning theorem” derives from Heisenberg’s Uncertainty Principle and dictates that a hacker cannot intercept or try to change one of the photons without altering them. The sharing parties will realize they’ve been hacked when the photon-encrypted keys no longer match.25
Another option looks to the cryptographic past while leveraging the quantum future. A “one-time pad” system widely deployed during World War II generates a randomly numbered private key that is used only to encrypt a message. The receiver of the message uses the only other copy of the matching one-time pad (the shared secret) to decrypt the message. Historically, it has been challenging to get the other copy of the pad to the receiver. Today, the photonic-perfect quantum communication channel described above can facilitate the key exchange. In fact, it can generate the pad on the spot during an exchange.
NOW WHAT?
We don’t know if it will be five, 10, or 20 years before efficient and scalable quantum computers fall into the hands of a rogue government or a black hat hacker. In fact, it’s more likely that instead of the general-purpose quantum computer, special-purpose quantum machines will emerge sooner for this purpose. We also don’t know how long it will take the cryptography community to develop—and prove—an encryption scheme that will be impervious to Shor’s algorithm.
In the meantime, consider shifting from asymmetric encryption to symmetric. Given the vulnerability of asymmetric encryption to quantum hacking, transitioning to a symmetric encryption scheme with shared keys and forward secrecy may help mitigate some “quantum risk.” Also, seek opportunities to collaborate with others within your industry, with cybersecurity vendors, and with start-ups to create new encryption systems that meet your company’s unique needs. Leading practices for such collaborations include developing a new algorithm, making it available for peer review, and sharing results with experts in the field to prove it is effective. No matter what strategy you choose, start now. It could take a decade or more to develop viable solutions, prototype and test them, and then deploy and standardize them across the enterprise. By then, quantum computing attacks could have permanently disabled your organization.
A view from the quantum trenches
Shihan Sajeed holds a Ph.D. in quantum information science. His research focuses on the emerging fields of quantum key distribution systems (QKD), security analyses on practical QKD, and quantum non-locality. As part of this research, Dr. Sajeed hacks into systems during security evaluations to try to find and exploit vulnerabilities in practical quantum encryption.
Dr. Sajeed sees a flaw in the way many people plan to respond to the quantum computing threat. Because it could be a decade or longer before a general-purpose quantum computer emerges, few feel any urgency to take action. “They think, ‘Today my data is secure, in flight and at rest. I know there will eventually be a quantum computer, and when that day comes, I will change over to a quantum-resistant encryption scheme to protect new data. And then, I’ll begin methodically converting legacy data to the new scheme,’” Dr. Sajeed says. “That is a fine plan if you think that you can switch to quantum encryption overnight—which I do not—and unless an adversary has been intercepting and copying your data over the last five years. In that case, the day the first quantum computer goes live, your legacy data becomes clear text.”
A variety of quantum cryptography solutions available today can help address future legacy data challenges. “Be aware that the technology of quantum encryption, like any emerging technology, still has vulnerabilities and there is room for improvement,”Dr. Sajeed says. “But if implemented properly, this technology can make it impossible for a hacker to steal information without alerting the communicating parties that they are being hacked.”
Dr. Sajeed cautions that the journey to achieve a reliable implementation of quantum encryption takes longer than many people think. “There’s math to prove and new technologies to roll out, which won’t happen overnight,” he says. “Bottom line: The time to begin responding to quantum’s threat is now.”26
Risk implications
Some think it is paradoxical to talk about risk and innovation in the same breath, but coupling those capabilities is crucial when applying new technologies to your business. In the same way that developers don’t typically reinvent the user interface each time they develop an application, there are foundational rules of risk management that, when applied to technology innovation, can both facilitate and even accelerate development rather than hinder it. For example, having common code for core services such as access to applications, logging and monitoring, and data handling can provide a consistent way for developers to build applications without reinventing the wheel each time. To that end, organizations can accelerate the path to innovation by developing guiding principles for risk, as well as developing a common library of modularized capabilities for reuse.
Once you remove the burden of critical and common risks, you can turn your attention to those that are unique to your innovation. You should evaluate the new attack vectors the innovation could introduce, group and quantify them, then determine which risks are truly relevant to you and your customers. Finally, decide which you will address, which you can transfer, and which may be outside your scope. By consciously embracing and managing risks, you actually may move faster in scaling your project and going to market.
Artificial general intelligence. AGI is like a virtual human employee that can learn, make decisions, and understand things. You should think about how you can protect that worker from hackers, as well as put controls in place to help it understand the concepts of security and risk. You should program your AGI to learn and comprehend how to secure data, hardware, and systems.
AGI’s real-time analytics could offer tremendous value, however, when incorporated into a risk management strategy. Today, risk detection typically occurs through analytics that could take days or weeks to complete. leaving your system open to similar risks until the system is updated to prevent it from happening again.
With AGI, however, it may be possible to automate and accelerate threat detection and analysis. Then notification of the event and the response can escalate to the right level of analyst to verify the response and speed the action to deflect the threat—in real time.
Quantum computing and encryption. The current Advanced Encryption Standard (AES) has been in place for more than 40 years. In that time, some have estimated that even the most powerful devices and platforms would take decades to break AES with a 256-bit key. Now, as quantum computing allows higher-level computing in a shorter amount of time, it could be possible to break the codes currently protecting networks and data.
Possible solutions may include generating a larger key size or creating a more robust algorithm that is more computing-intensive to decrypt. However, such options could overburden your existing computing systems, which may not have the power to complete these complex encryption functions.
The good news is that quantum computing also could have the power to create new algorithms that are more difficult and computing-intensive to decrypt. For now, quantum computing is primarily still in the experimental stage, and there is time to consider designing quantum-specialized algorithms to protect the data that would be most vulnerable to a quantum-level attack.
BOTTOM LINE
Though the promise—and potential challenge—exponential innovations such as AGI and quantum encryption hold for business is not yet fully defined, there are steps companies can take in the near term to lay the groundwork for their eventual arrival. As with other emerging technologies, exponentials often offer competitive opportunities in adjacent innovation and early adoption. CIO, CTOs, and other executives can and should begin exploring exponentials’ possibilities today.
Authors
Jeff Margolies is a principal with Deloitte and Touche LLP’s Cyber Risk Services practice, based in Los Angeles.
Rajeev Ronanki leads Deloitte Consulting LLP’s Cognitive Computing and Health Care Innovation practices and is based in Los Angeles.
David Steier is a managing director for Deloitte Analytics with Deloitte Consulting LLP’s US Human Capital practice, based in San Jose, Calif.
Geoff Tuff is a principal with Deloitte Digital and is based in Boston.
Mark White is the chief technologist for the US innovation office with Deloitte Consulting LLP, based in San Jose, Calif.
Ayan Bhattacharya is a specialist leader with Deloitte Consulting LLP and is based in Philadelphia.
Nipun Gupta is a senior consultant with Deloitte and Touche LLP’s Cyber Risk Advisory practice, based in San Francisco.
Irfan Saif is an advisory principal with Deloitte and Touche LLP and is based in San Jose, Calif.
9. Cảm ơn Tác giả
Excutive Editor
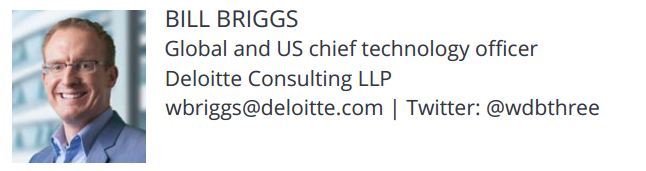
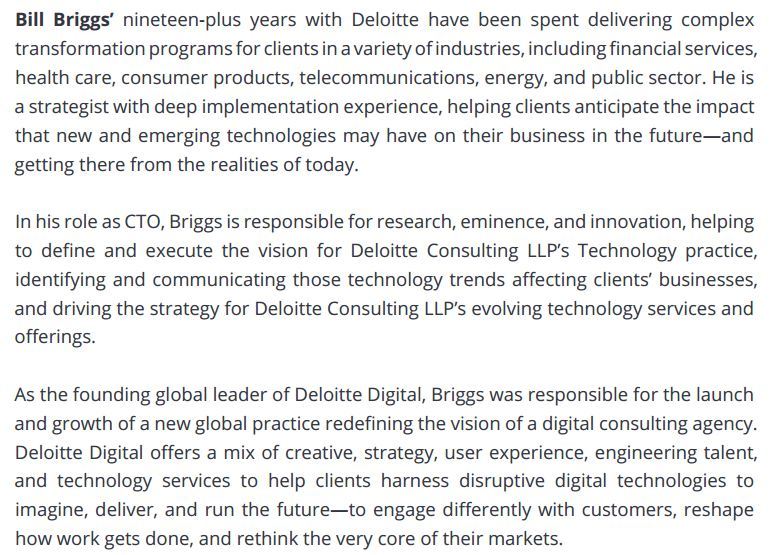
Global Impact Authors
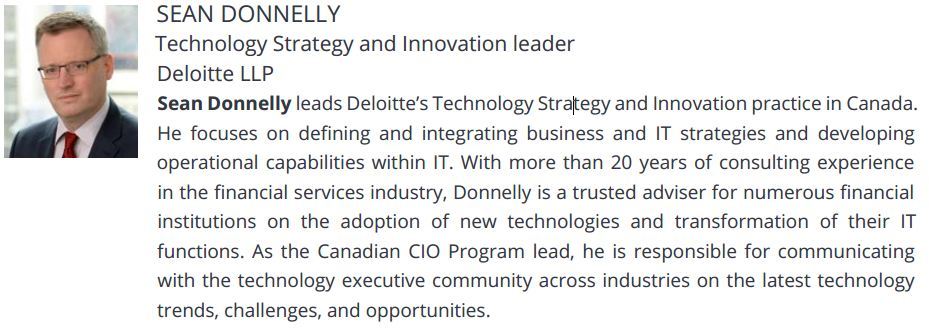
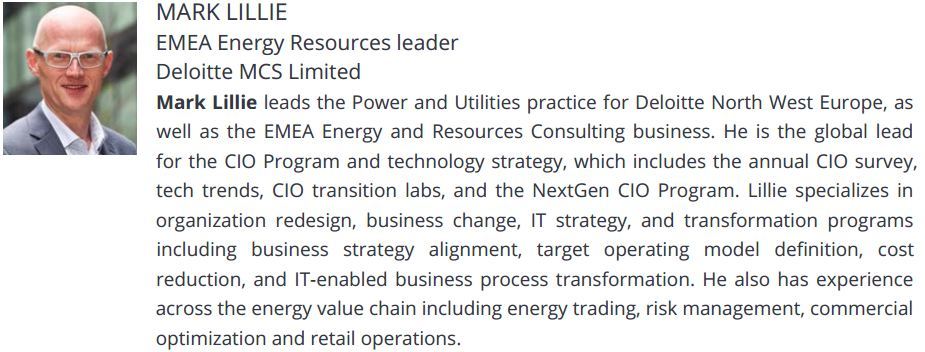
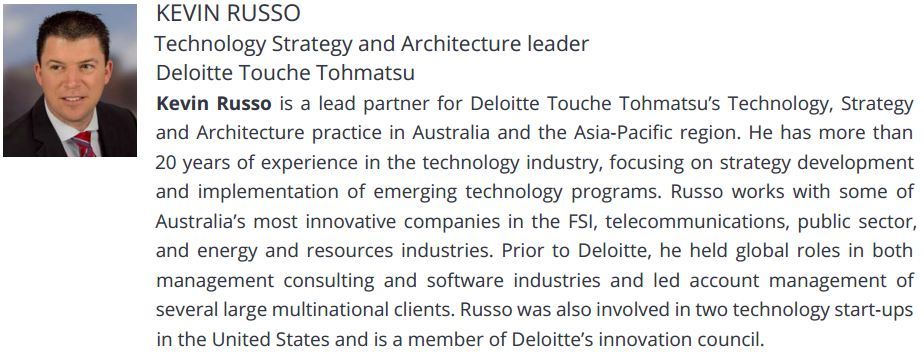
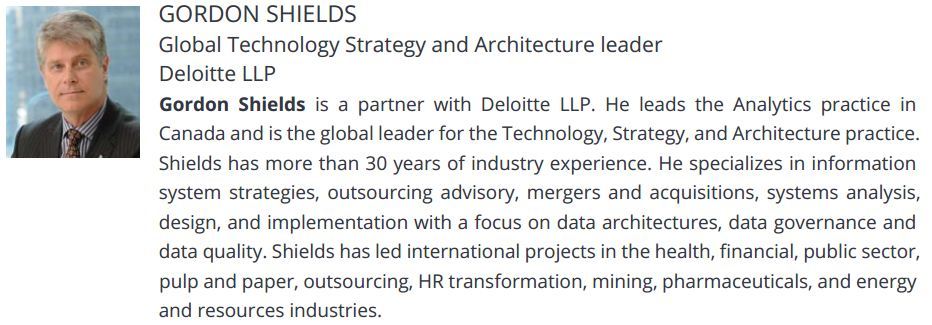
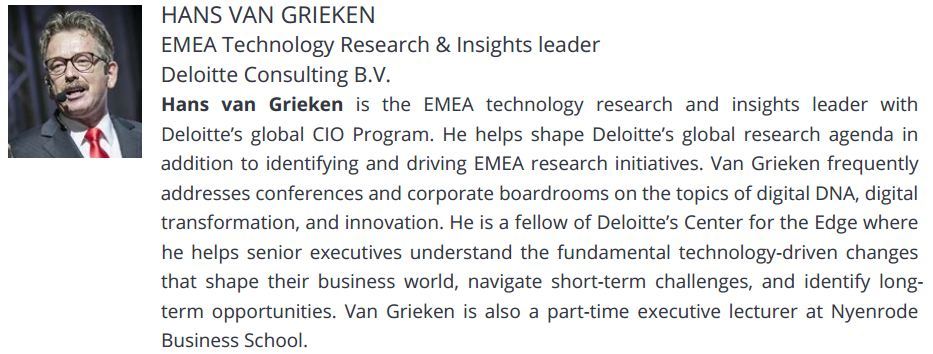
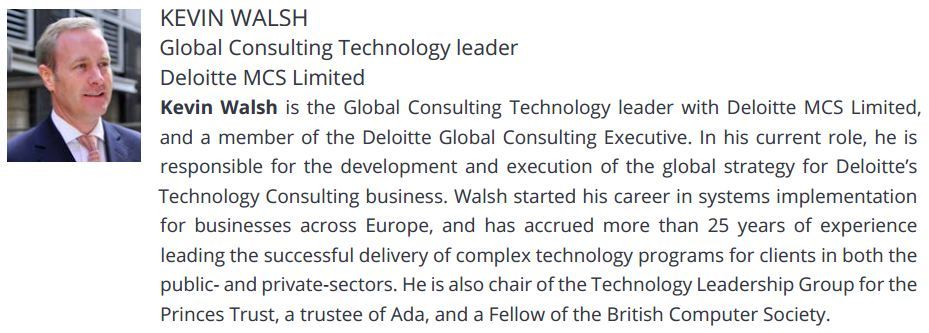
Cộng tác với
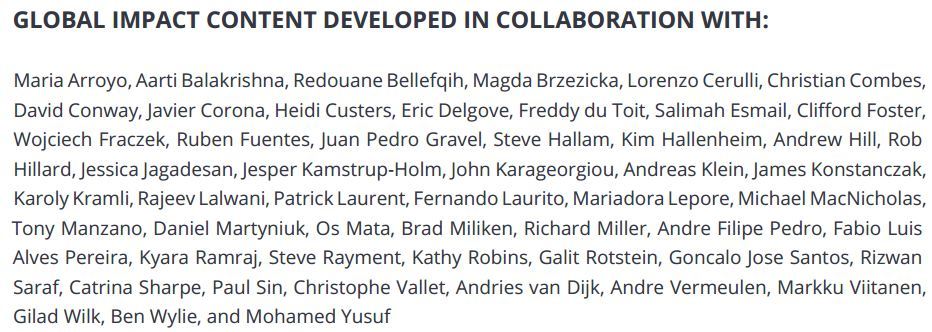
Đóng góp bởi
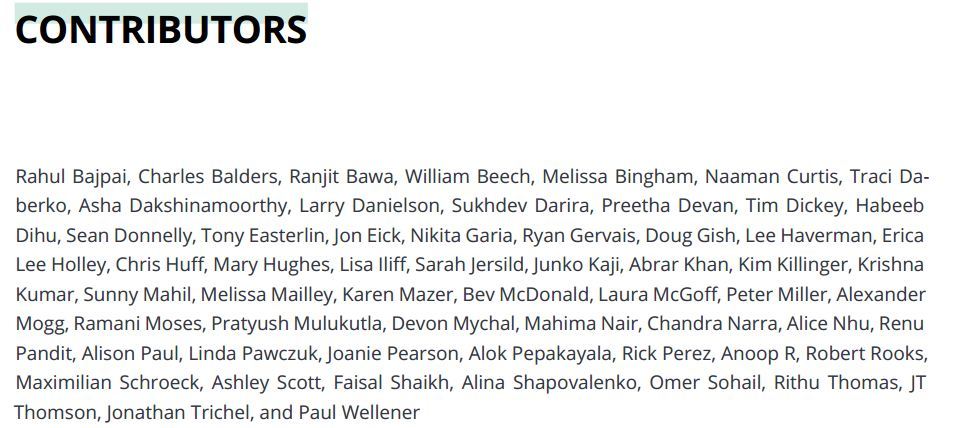
Nhóm nghiên cứu
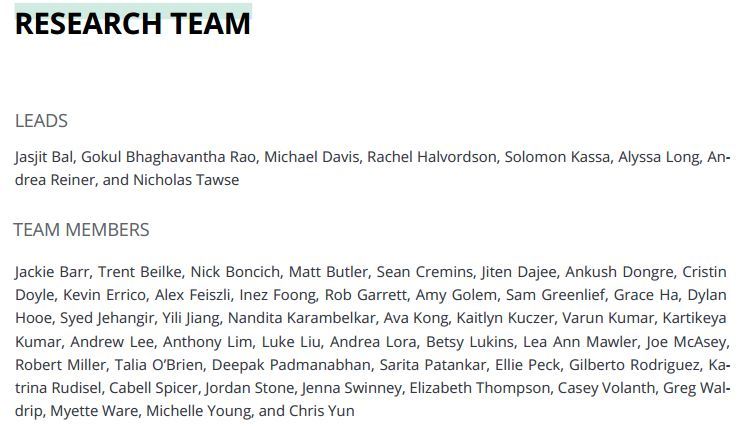
Lời cảm ơn Đặc biệt
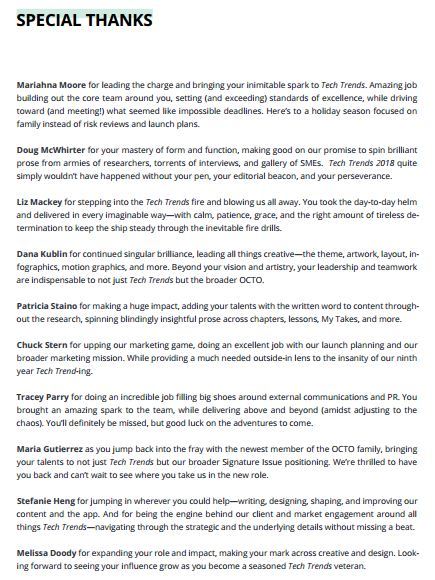
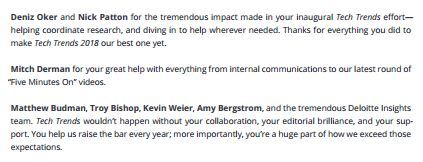
Thử nghiệm Donate
Nếu thấy hay thì có thể Donate cho tác giả bằng cách chuyển khoản và để lại tin nhắn theo cú pháp:
Donation - Tên người gửi tiền - Người suy nghĩ - Lời nhắn.
Trần Việt Anh
STK: 0451000364912, ngân hàng Vietcombank chi nhánh Thành Công, Hà Nội
Cảm ơn đã đọc, và nhớ Donate !

Khoa học - Công nghệ
/khoa-hoc-cong-nghe
Bài viết nổi bật khác
- Hot nhất
- Mới nhất
Hãy là người đầu tiên bình luận bài viết này